In today’s dynamic business environment, efficient resource allocation and planning are paramount for the success of any organization. Microsoft Dynamics 365 Customer Service brings a powerful forecasting feature that empowers businesses to predict and manage their service volumes and agent demands effectively. Leveraging AI-based algorithms, this intelligent forecasting model runs behind the scenes, analyzing historical data and trends to provide accurate predictions. With the ability to forecast both volume and agent demand, organizations can streamline their operations, optimize resource allocation, and ultimately enhance customer satisfaction. This blog post focuses on volume forecasting for cases and conversations as well as agent forecasting for conversations.
Front-office and back-office forecasting
Dynamics 365 Customer Service recognizes the diverse nature of service channels, distinguishing between front office and back-office operations. Front office channels encompass voice, chat, email, messaging, and social channels, representing direct interactions with customers referred to as conversations in Dynamics 365. Back-office operations, on the other hand, refer to cases that require internal processing and resolution. Customer Service offers flexibility in forecasting front-office, back-office, or blended agents. This capability enables organizations to tailor their strategies to the specific needs of each operational area, ensuring optimal resource utilization and service delivery.
Volume forecasting
Forecasting provides daily forecasts for case and conversation volumes for up to six months into the future. Daily forecasts enable organizations to anticipate and prepare for fluctuations in service demand. Additionally, the system offers intraday forecasts at 15-minute intervals. Intraday forecasts allow for granular planning up to six weeks ahead. This level of foresight empowers businesses to allocate resources efficiently, ensuring optimal service levels.
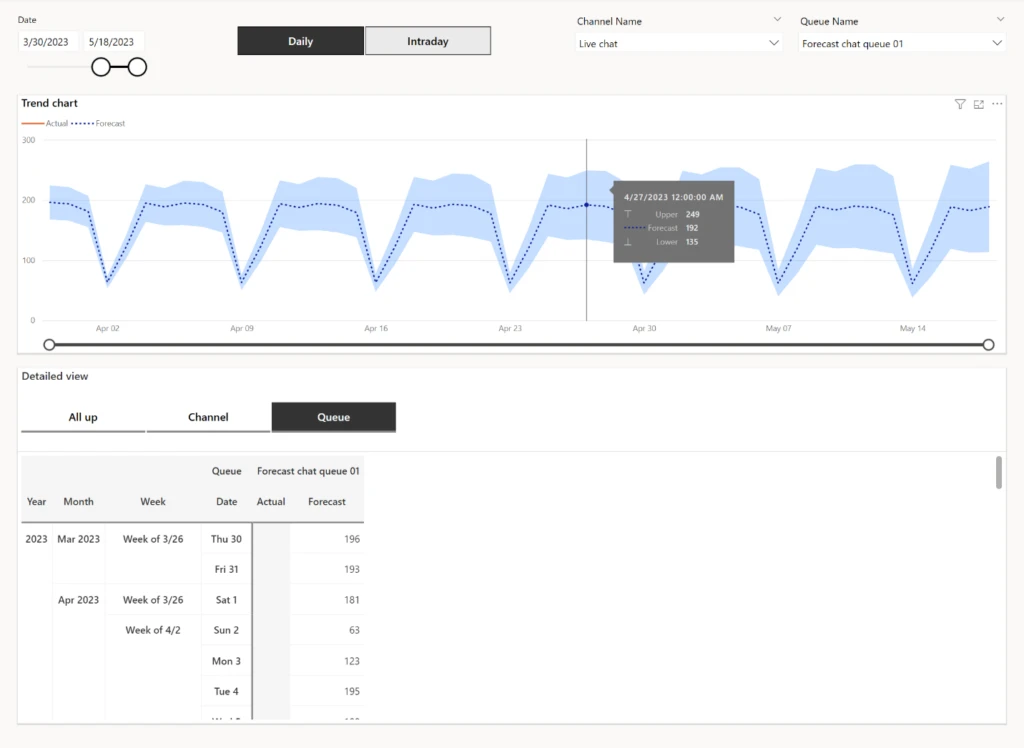
Agent Forecasting
In addition to volume forecasting, organizations can forecast agent demand for conversations on a daily interval for up to six months into the future. Like volume forecasting, the system provides intraday forecasts at 15-minute intervals, allowing for precise resource allocation and scheduling.
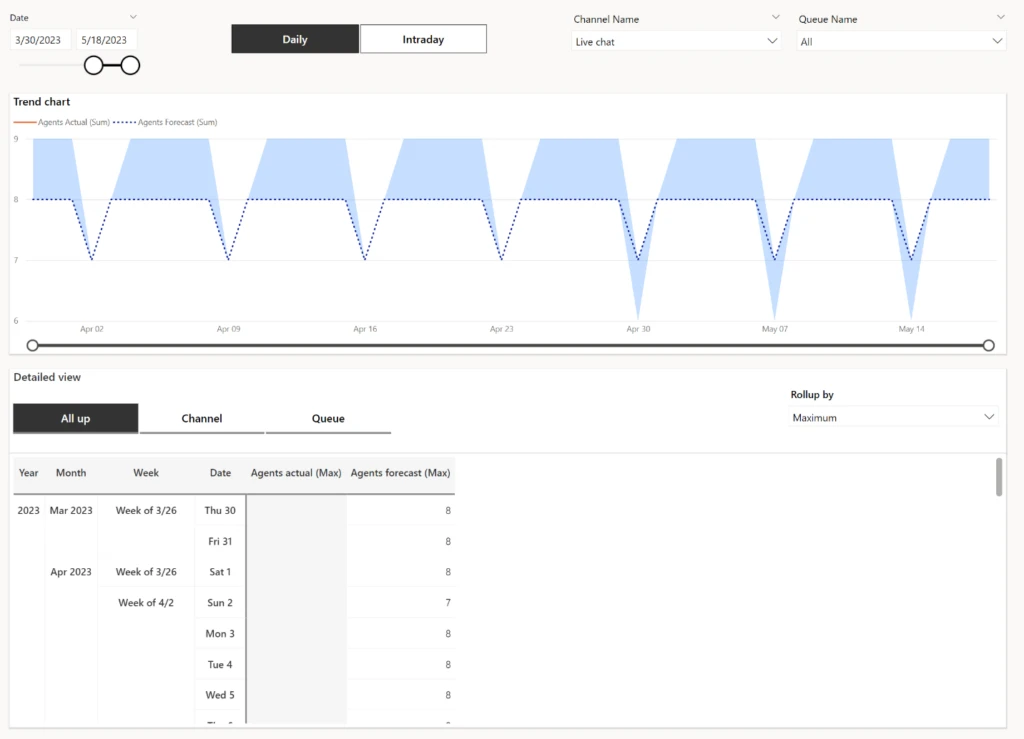
Incorporating service-level metrics
The feature considers operational metrics such as service level, shrinkage, and concurrency when forecasting agent demand. By considering these factors, organizations can ensure that the agent capacity forecast aligns with service level agreements and operational constraints, maximizing efficiency and customer satisfaction.
Auto-detection of seasonality
By analyzing historical traffic patterns, our AI model automatically detects seasonality, enabling more accurate forecasts. This feature helps organizations adapt their operations to seasonal variations in service demand. Addressing these variations helps organizations maintain high service levels regardless of fluctuations in customer activity.
Auto-detection of holidays
Our forecasting model utilizes historical traffic patterns to automatically identify holidays, which leads to more precise predictions. This functionality assists organizations in adjusting their operations according to holiday-related changes in service demand across various regions, guaranteeing that they can maintain optimal service levels despite fluctuations in customer activity during holidays.
Forecast vs. actual charts
User-friendly charts are available to visually represent service volume and agent demand forecasts alongside actual performance across daily, weekly, and monthly intervals for up to six months. This comparison enables organizations to assess the accuracy of their forecasts and identify areas for improvement.
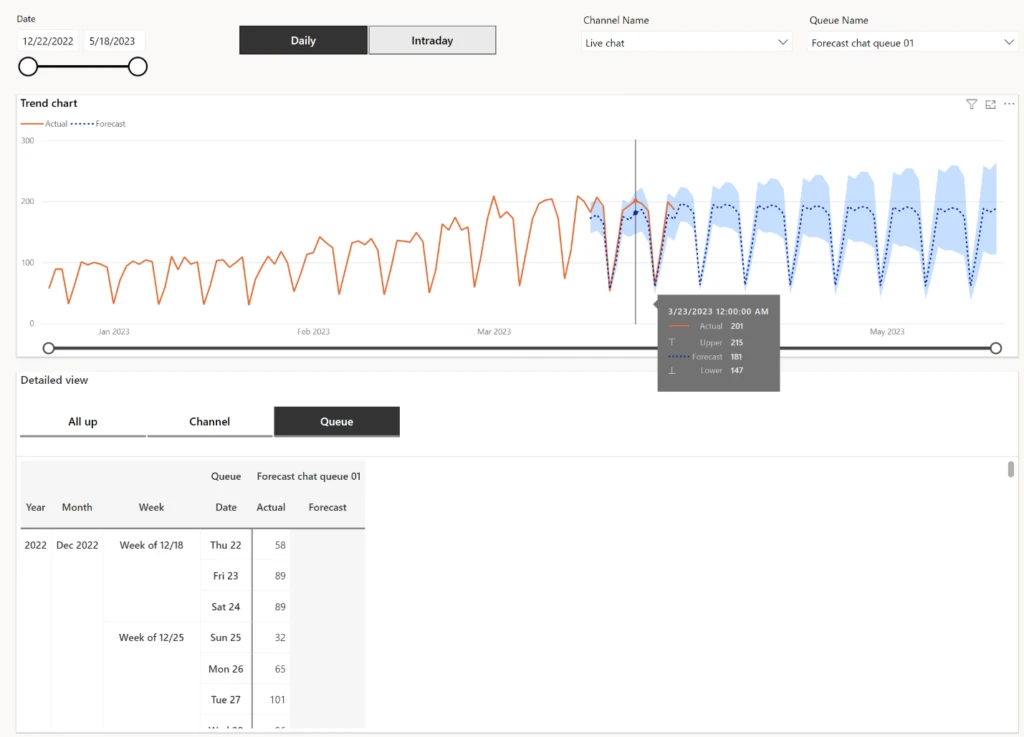
Customizable slicing and exporting
The flexibility of forecasting extends to its ability to slice forecast data by channels and queues, providing insights tailored to specific operational needs. Moreover, users can export forecast data into a spreadsheet for further analysis or integration with other tools, enhancing the usability and accessibility of the forecasting feature.
Key considerations for accuracy
We recommend the following criteria for using historical data to generate accurate forecasts.
Non-sparse data: The dataset contains information for every day, ensuring that there isn’t missing or incomplete data. Each day has a recorded volume, providing a comprehensive set of observations.
Clear weekly pattern: The data shows a weekly pattern, wherein the volume consistently follows a specific trend. For instance, weekends consistently have low volumes, while workdays show higher volumes, and vice versa. This pattern helps establish a reliable basis for forecasting.
Volume-based accuracy: If the criteria are met, the forecast quality improves with larger volume inputs. Higher volumes of data contribute to a more accurate and robust forecast.
Absence of level shift: Recent days and future periods don’t experience any sudden or significant shifts in volume levels. This absence of sudden changes ensures that the historical patterns stay relevant and dependable for forecasting purposes.
Longer historical data set: If all the above criteria are met, a longer history of data further improves the forecast accuracy. A greater historical data set provides a more comprehensive understanding of the patterns and trends over time. With an extended history, the forecast model can capture and incorporate more variations, leading to more accurate predictions.
Weighting recent forecast accuracy: When considering future periods, understand the forecast’s accuracy tends to be higher for more immediate timeframes. As time progresses, the certainty and precision of the forecast may decrease. Therefore, the most recent forecast should be given more weight and considered to have better accuracy compared to future forecasts.
Stay ahead of the curve
In conclusion, forecasting offers a comprehensive solution for predicting support volumes and agent demand, empowering organizations to optimize their operations and enhance customer satisfaction. With daily and intraday forecasts, and auto-detection of seasonality, businesses can achieve greater efficiency and agility in their service delivery. With Dynamics 365 Customer Service, organizations are driving success and growth through effective resource planning and management.
Learn more about forecasting
To learn more about forecasting, read the documentation: Forecast agent, case, and conversation volumes in Customer Service | Microsoft Learn
The post Maximize efficiency with forecasting in Dynamics 365 Customer Service appeared first on Microsoft Dynamics 365 Blog.